Assimilative Model Assessment of Pioneer Array Data
Adapted and condensed by OOI from Levin et al., 2020, doi:/10.1016/j.ocemod.2020.101721.
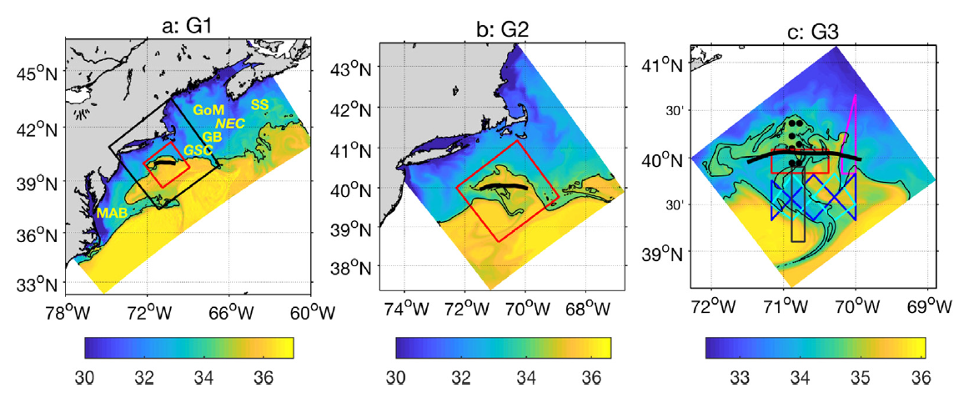
Fig. 1. Color contours of sea surface salinity from the model for three nested grids denoted a) G1, b) G2 (black square in (a)) and c) G3 (red square in (a), (b)). An indicator of frontal position (the 34.5 isohaline) is shown as a black contour. Cross-shelf exchange parameters are computed for an along-shelf section (thick black line). The Pioneer Array assets are shown in the G3 figure.
Among the detailed analyses undertaken in this two-part study was quantification of the impact of observations on the reduction of RMS error for estimates of the volume transport across an along-front transect (Fig. 1). Temperature and salinity data from moorings and gliders were impactful for the larger grids (G1, G2). As the grid resolution was increased (G3), submesoscale motions were resolved and velocity data from the moorings became more important for reduction of error variance. An analysis of the sensitivity of shelf-slope exchange indices (e.g. volume transport) to removal of an observation, compared to the direct impact of the observation, showed that the majority of observed variables (e.g., SST, SSH, T, S, U, V) were “synergistic” – providing value to the assimilation through their connection with other variables as represented in the model dynamics. For the highest resolution estimates (G3 grid), the Pioneer Array observing assets were more impactful than other observations (e.g., remote sensing, NDBC and IOOS buoys) in reducing uncertainty, with velocity data being the major contributor. This is not a complete surprise, since the Pioneer Array was “tuned” to these scales. Still, it is gratifying to see that the impact on model fidelity is quantifiable.
The two-part study undertaken by Levin et al. provides a wealth of additional information about the performance of assimilative models as well as the utility of in-situ observations for modeling and prediction. As the authors state, they have “just begun to scratch the surface” of approaches that can be applied to the assessment of model performance as well as the management of observing systems.
Levin J., H.G. Arango, B. Laughlin, E. Hunter, J. Wilkin, and A.M. Moore, 2020. Observation impacts on the Mid-Atlantic Bight front and cross-shelf transport in 4D-Var ocean state estimates: Part I – Multiplatform analysis,Ocean Modeling, 156, 101721, 1-17, doi 10.1016/j.ocemod.2020.101721.